“Now, what I want is Facts”, says Thomas Gradgrind in the opening line of Charles Dickens’ Hard Times. Replace the word “Facts” with “Data” and aficionados of big data will readily agree. Some even assert there will be no more need for theory because data patterns already tell us the whole story. Are such claims ill-founded? After all, scientists have been working with data for centuries. Empirical data have been the linchpin of the social and natural sciences ever since the latter emerged to supersede what was increasingly felt to be unempirical scholastic quibbling.
This article explores the validity of claims that theory has lost its practical purpose in a world where the abundance, and easy availability, of data is purported to provide sufficient guidance to our actions. In a future Risk Insights article we will consider the possible consequences for the life insurance industry with a particular eye on the future role of human expert knowledge.
The end of theory
The last person to proclaim the end of something momentous was the American political scientist Francis Fukuyama. In 1989 he argued that history was about to culminate in a final stage in which the entire world would be governed by liberal democracies.1 Alas, history, far from coming to a standstill, has proved him wrong.
But do data aggregators, such as Google, represent the gravediggers of theory? Is it “time to ask: What can science learn from Google?”2 Or do aficionados of big data succumb to its undisputable momentum in the same way Fukuyama was overwhelmed by the epochal political changes in the early 1990s?
The idea that big data will allow direct empirical access to the world, stripped of any theory, has been discussed repeatedly in recent years.3 In reality it is simply a recent manifestation of an age-old dispute in the history of science and philosophy. Prominent rationalist philosophers, among them Descartes and Leibniz, claimed that certain knowledge could only result from reason and logic. Conversely, the empiricist school of Bacon, Locke and Hume held that experience formed the ultimate foundation of knowledge. Bacon went as far as to assert that empirical facts would speak for themselves.4
The foundation of knowledge became a matter of dispute again in the first half of the 20th century, opposing the followers of “falsificationism” with the advocates of “logical positivism”. This time it was scientific knowledge that was at stake, or more precisely, the criteria a knowledge claim must obey in order to qualify as scientific.
According to the philosopher Karl Popper, scientific knowledge claims (theories) must, in principle, be refutable (falsifiable) on the basis of empirical observation.5 For example, the theory that says, “It will rain tomorrow” is scientific because it will be refuted if it does not rain tomorrow. On the other hand, the theory that says, “It will, or will not, rain tomorrow” is unscientific, since no conceivable observation statements could refute the theory.
Logical positivists held the view that the essence of scientific knowledge was rooted in basic empirical observation statements and that its inductive method was a defining element. On this basis, the claim (theory) that it will rain tomorrow can only count as scientific if it has already rained for many days. Otherwise, it would have to be considered as unscientific guesswork.
Do we need knowledge?
Promoters of big data assert that knowledge is no longer required to operate successfully in a digital world. In their words “correlation [a series of patterns] supersedes causation [read: knowledge], and science can advance even without different models, unified theories, or really any mechanistic explanation at all.”6
This view echoes the scepticism inherent in the empiricist school of thought regarding the timeless certainty of knowledge. Consider Figure 1, which depicts a web of mutually related pieces of information (I), and in which each piece of information accounts for other pieces of information (blue arrows). This network constitutes knowledge. Without the explanations and accounts that relate one piece of information to the other, we are left with a pile of bits of information, which “cannot help to make sense of the reality they seek to address.”7
Information can be described as the sum of data (D) and meaning (M), i.e. I = D+M.8 Advocates of big data claim that knowledge can be dispensed with completely because “meaning” may be subtracted from this equation. Well-formed data put together according to certain rules (syntax) are sufficient – as in translations or advertising – with no need for any information about languages, culture and conventions.
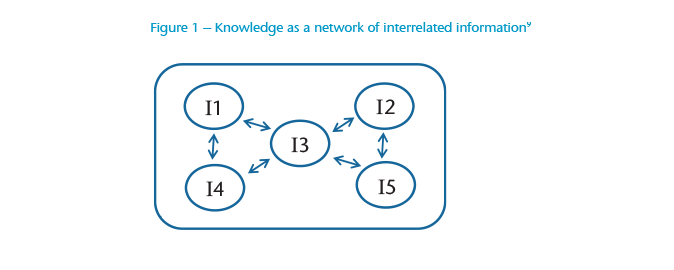
To assess if knowledge really is no longer needed, we must consider the heuristic distinction between specific knowledge and background knowledge.10 Specific knowledge encompasses the explanations that render statistical correlations plausible in the light of our background knowledge. Background knowledge, apart from supporting specific knowledge, enables us to collect data in a target-oriented manner and to sift the valuable data patterns.11 Moreover, it helps us exploit the correlations we have detected and, possibly, explained. The first makes our predictions more reliable while the second makes them more profitable, and not only in a commercial sense.
An example from the history of psychology serves to show the benefits of specific knowledge. In the first half of the last century, a theoretical approach known as “behaviourism” became influential (Box 1).
Box 1 – Behaviourism
Unlike the Freudian psychodynamic approach with its focus on (unobservable) subconscious mental processes, behaviourism was seen by its proponents as “a purely objective branch of natural science.”12 Its theoretical objective was “the prediction and control of behaviour.”13 The behavioural theory was fully developed by B.F. Skinner, who supplemented the earlier focus on classical conditioning, i.e. stimulus provokes response, by so-called operant conditioning, i.e. accidental behaviour is reinforced or punished by an operant, and, as a result, the likelihood of such behaviour either increases or decreases. According to the behavioural theory this was the essence of learning. Skinner did not deny the existence of mental states accompanying operant conditioning, but he claimed that they would be irrelevant (see Figure 2).
Behaviourism, in its refusal to explain the correlation between observable operants and observable behaviour, anticipates big data’s rejection of knowledge. Its validity was subsequently challenged by another theoretical approach, called cognitive psychology.
Cognitive psychology asserts that mental states and the way the mind operates do matter. In an experiment, participants were asked to report their true opinions on certain topics and subsequently to contradict these opinions in short essays in return for money in varying amounts.14 In a final step, they had to complete questionnaires, which they were told would be anonymous, in order to collect their true opinion again.
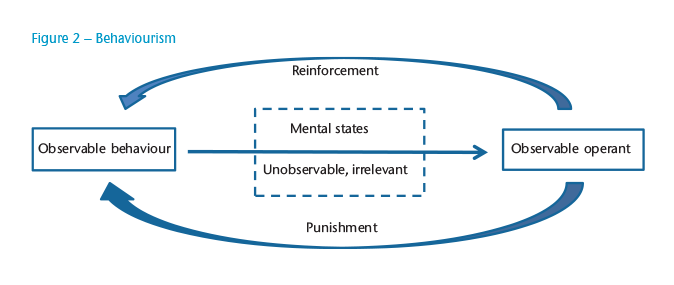
Behaviourists would expect that among test subjects given higher amounts of money for contradicting their initial opinions (reinforcement), a higher proportion would report a greater change in opinion in the final questionnaire, i.e. they learned the new opinion. Actually, the opposite occurred: The smaller the reward, the greater the change in opinion induced by the experiment.15
Box 2 – Cognitive Dissonance
According to the theory of cognitive dissonance, conflicts in the mind between incompatible opinions or between opinions and behaviour will induce subjects to either change their opinion or adapt their behaviour so that peace of mind will be restored. This process also depends on how well a new conflicting opinion or behaviour can be justified (the amount of money in the above experiment). Poor justification (e.g. less money reward) means more psychological disturbance (cognitive dissonance) and, consequently, a higher propensity to change behaviour or opinion.
This outcome was correctly predicted by cognitive psychology, or more precisely the theory of cognitive dissonance (Box 2). Students with a smaller reward were more affected by their conflicting behaviour and, therefore, felt a greater urge to change their opinion in order to bring it in line with their previous behaviour. Figure 3 illustrates the fact that theory can be relevant in order to explain (observable) correlation.
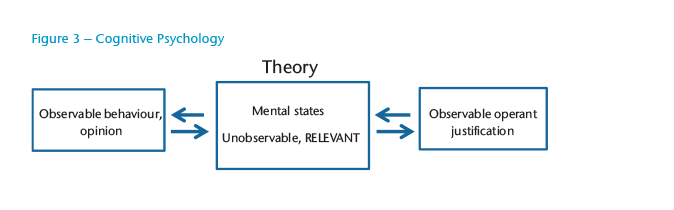
The benefits of having knowledge
The benefits of background knowledge become apparent in the following example.16 Data analysts, employed by Target, an American retailing company, had found out that the purchasing patterns of 25 products enabled them to assign pregnancy prediction scores along with estimations of due dates. Target knew, having consulted neurological and psychological insights on habit formation (notably the loop cue, routine and reward), that people were receptive to habit-changing cues upon the occurrence of major life events, such as pregnancy.
Target launched tailor-made adverts for baby-related products to entice pregnant women and their relatives to visit their shops. There they would be pushed to buy other products in a bid to change their purchasing habits. But there was a potential public relations disaster looming, which Target was able to spot in time due to their cultural and psychological background knowledge.
The company realized pregnant women might be alarmed by tailor-made adverts for pregnancy-related products. These women could rightly have wondered how Target had come to know about their pregnancy or even whether they were being spied on. Target therefore started mixing in adverts for products pregnant women might never buy, such as lawn mowers or wine glasses.
Conclusion
There appears to be no reasonable doubt that background knowledge will remain valuable in a digital world governed by big data. We still need to ask the right questions and this is impossible without background knowledge. big data aficionados will most likely agree. Their scepticism is rather directed at specific knowledge in the form of theories explaining statistical observations, notably correlations. This knowledge, according to them, was necessary in the past in order to boost our confidence in predictions, which were based on a limited number of observations (data). Theory was merely an auxiliary device and could not claim any higher dignity. With the advent of big data, sampling, inductive inference and hypotheses are being replaced by the real-time observation and tracking of entire populations.17 Theory seems no longer necessary to support the inductive leap from a limited number of observations to the target population in question. For commercial applications, this appears to be a valid view, especially in view of the speed with which errors can be corrected at practically no cost. Nevertheless, pitfalls remain, as the above example from a behavioural psychology experiment shows.
A brief look at possible definitions of the term “big data” mirrors most of the controversial points we have discussed. One interpretation is it refers to a data set that has become too large to be managed with traditional database techniques.18 Volume plays an additional role in the sense that big data applications do not need to sample but can simply observe and track what happens.19 In another view, big data is not so much about the lack of computational power but about the detection of new patterns, correlations that can provide added value.20 Velocity is, next to volume and variety, another important characteristic of big data, as calculations are usually made in real time.21
In a future Risk Insights article we will highlight characteristics of big data that can truly be considered as new and transformative, and we will examine the additional impetus generated by accelerating social change. The focus will shift to practical (ethical) questions surrounding the use of big data. To conclude, we will review how big data has already influenced and will further shape the way life insurers tackle their major challenges, i.e. customer needs, risk of change, anti-selection and moral hazard.